Why Visualization is Challenged?
Learn about the various challenges that come with the visualization process.
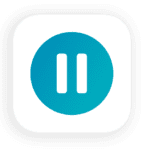
Selfpause Affirmation App
Download the app to get 1,000’s of affirmation meditations and everything you need to write, record and listen to your own.
Visualization is becoming a popular way to communicate with consumers. They use imagery to draw conclusions and are easy to absorb. Visuals are likely to remain relevant as technology advances. However, data visualization is not without its challenges. Here are some of them. Visualization is important for staying competitive in a fast-paced world.
Challenges of data visualization
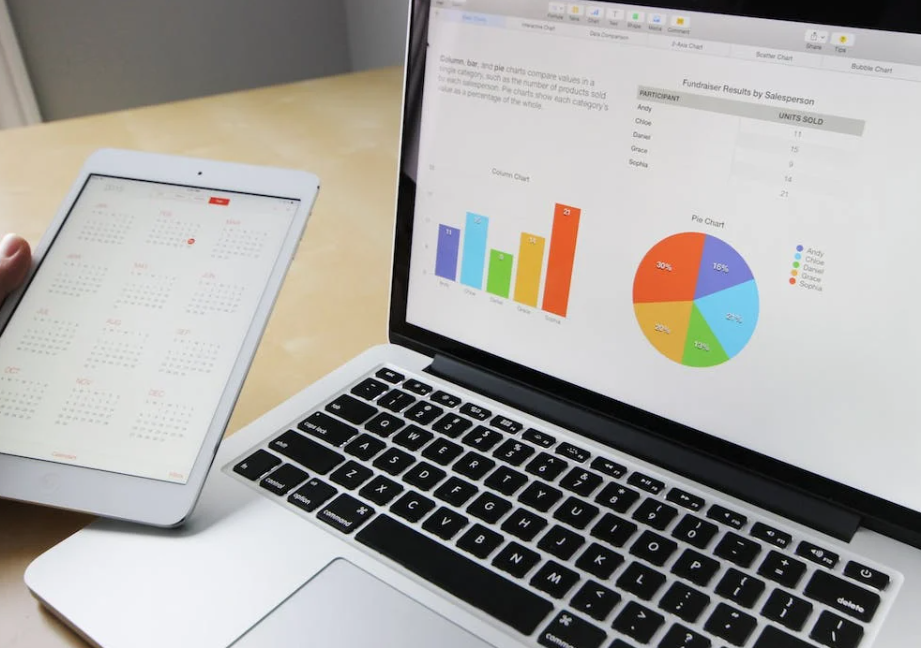
There are many challenges involved in the process of data visualization. Creating a good visualization requires a lot of information, which may be difficult to obtain. The data must also be in an accessible format and licensed for reuse. Finally, the data must be available in real-time. These challenges make data management an important part of the process. However, this process does not happen overnight, and there are several steps that need to be followed before the data is ready to be visualized.
Data visualization helps us make sense of the data that we collect every day. By removing noise and highlighting useful information, good visualizations allow us to understand the data better. In order to create a good visualization, the data and the visuals must be harmoniously combined. The data visualization process requires great analysis combined with good storytelling.
Creating a data visualization takes considerable time, money, and effort. Traditional data visualization tools required a high level of technical expertise and entailed hiring developers to maintain them. This approach was clunky and complex, and it required a lot of development time. But thanks to new self-service data visualization tools, even non-IT professionals can create beautiful visualizations.
Data visualization can help business users understand large sets of data by revealing hidden patterns. It can also uncover relationships that were previously invisible. By putting the data in an intuitive manner, users can focus on the areas that are important to them. This way, business users can focus on the most useful information while removing the noise.
Methods of data visualization
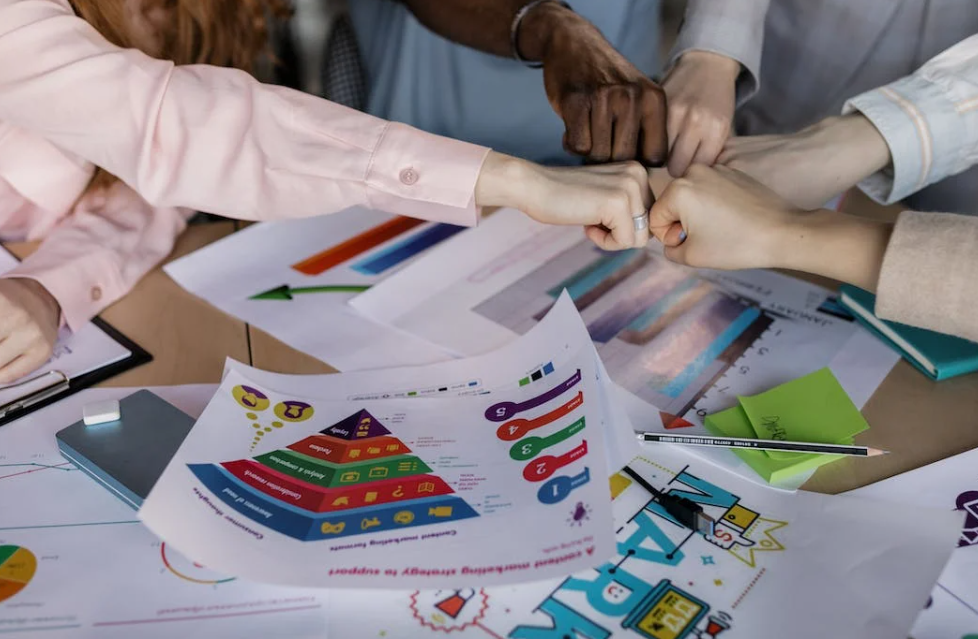
Data visualization is the process of observing and interpreting complex data. It is a key part of the cognitive process, leveraging human capabilities to process 10 million bits per second and recognize patterns within 100 milliseconds. Traditionally thought of as a luxury for scientists, it has recently become a necessary part of the research. Companies like Tableau and Looker have gone public, proving that the process of visualizing data is both powerful and useful.
Depending on the topic, data visualization can include a variety of methods, including pie charts, bar graphs, and line graphs. Using the right data visualization method can help your audience better understand the topic. The challenge of presenting data, however, is that there are no single, universal methods.
Modern data sets and data formats present a unique set of challenges. Not only must the visualization method account for non-i.d. data, but it must also deal with the fact that many of these data types have different levels of credibility. In addition, it is important to select the right dissimilarity measure for the dataset.
Data visualization is a critical part of the discovery process in today’s big data era. Effective visualization helps you make sense of vast amounts of data and discover new insights. For instance, the more dimensions you can see, the more likely you are to spot patterns. Another challenge is visual noise. This is caused by the close proximity of objects in the dataset. Visual noise also affects our ability to recognize patterns in data visualization.
There are many different methods of data visualization. For example, there are some methods of data visualization that are useful for business analytics, while others are more suitable for communicating complex ideas to a wider audience.
Challenges of integrating big data with visualization
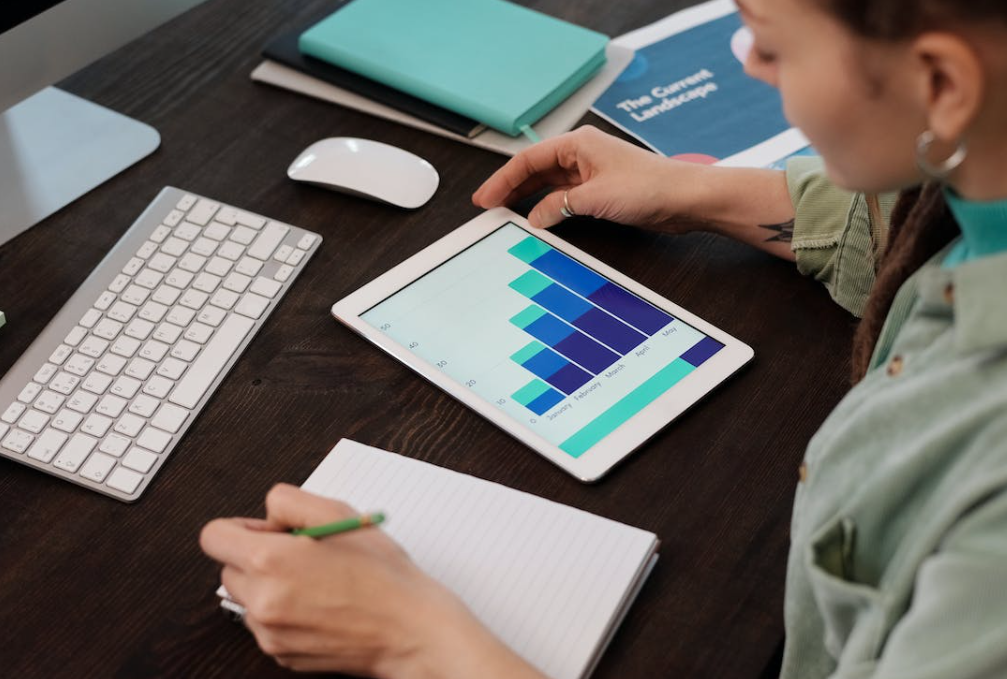
Visualization is a powerful tool for creating attractive infographics and business value from large data sets. These data are often unstructured, semi-structured, or both. It is important to index these data quickly and efficiently to facilitate analysis. The scalability of visualization is also an important consideration.
Data visualization offers tremendous opportunities for research and innovation. The high dimensionality and heterogeneity of the collected data present a number of challenges for visualization. These challenges can be overcome by designing data aggregation and visual composition strategies. Other challenges include improving computing scalability and addressing issues associated with data transformation and generation.
Visualization of big data requires proper domain expertise. It should provide a broad overview but also provide filtered and detailed information on demand. It should also address the relationship between data and the network, such as in social networks. The right visualization can highlight emerging trends in the network. An example of this is the visualization of inherence between users of social networks.
Large data sets only add value if they can be understood. Because they come from different sources, data analysis can be challenging and requires sophisticated analytical skills. Moreover, data is often time-sensitive. It may become obsolete in seconds. This puts tremendous pressure on data integration. As a result, a Forrester Consulting survey found that only 38% of respondents had confidence in the insights they derived from their big data.
Using visualization is crucial for the integration of big data. A good visualization can help make sense of data and drive business decisions. However, it should be integrated with data analysis tools. Tableau, for example, is an end-to-end data analytics tool that can make big data accessible to anyone. The software enables people to ask questions and perform a visual analysis of governed big data.
Importance of integration
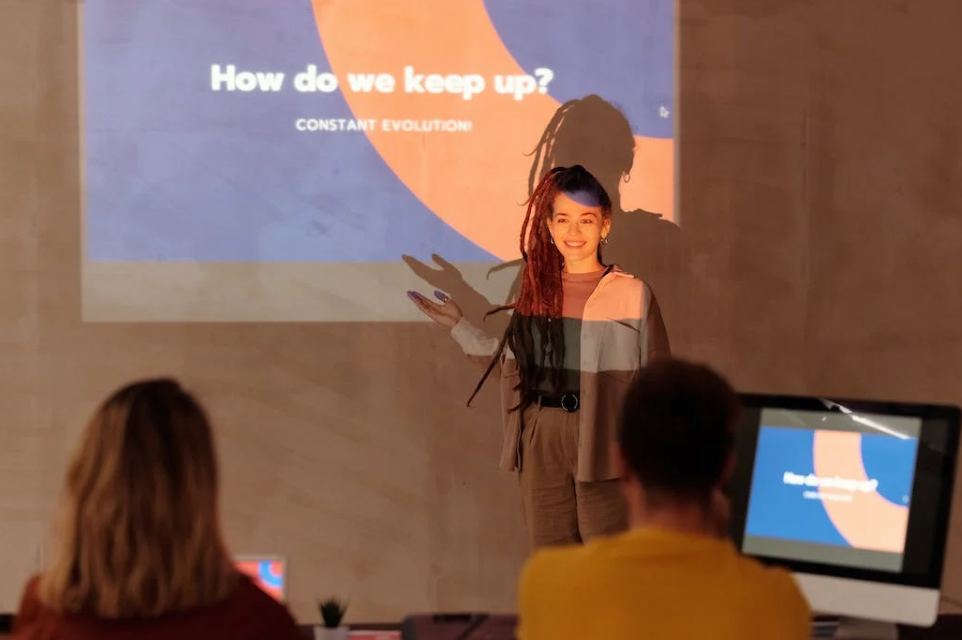
Integration in visualization is crucial for businesses to make the most of their data. With the ability to visually display vast amounts of data, organizations can easily identify trends and take action. This helps them increase profits and avoid loss. Visuals are easier for human beings to process than data presented in tabular form. Additionally, visual data can help identify errors and improve decision-making speed.
There are several factors to consider when planning an integration project. First of all, consider the timeframe. If you must integrate disparate data manually, it is imperative to determine how much time you’ll save. Second, you should consider the scalability of your system. Integration projects must be able to scale and adjust according to your business needs.
Data visualization tools must have built-in integration capabilities. These integration options allow them to easily load and merge data from various sources. This opens up endless possibilities for analysis. In the business world, data is one of the most important assets. This means that data integration is essential to keeping your data safe and accurate.
Integration is also critical for smart cities. Smart cities have thousands of sensors, each with different detection capabilities, which generate huge amounts of raw data. Such data helps make informed decisions and is crucial for accountability. It also allows users to easily understand and manipulate information. This makes data accessible to everyone and fosters a data-driven culture.
Importance of persuasion in data visualization

Persuasive data visualization can be effective in influencing people to make decisions and act on them. These visualizations may be data-driven or concept-driven. Students should be sure to choose the most appropriate method for their purposes. In addition to visual means of persuasion, they should also consider other forms of persuasion such as oral and written communication. One example is a project in which students design a one-page infographic with the help of peer feedback. The final product should be a persuasive, modular visualization that ties to the learner’s interest in advocacy and generalizes across disciplines.
Data visualization can be a powerful influencer, breaking through the clutter and telling a story. When paired with the right story, it can even persuade people to take action. Raw statistics are fine, but showing them in context is the future of information sharing. All communications professionals should take this into account when creating and sharing their communications.
The authors of this book are well-versed in the field of data visualization. They present essential tips to create compelling visualizations and explain how they work. Their goal is to help readers overcome information overload by identifying trends and patterns that are clear and unambiguous. Ultimately, data visualization can change the world.
Creating compelling data visualizations for internal stakeholders is not as difficult as it may seem. In fact, it’s easier than ever to share information thanks to the massive amount of data and tools available today. With the help of these tools, even managers can create compelling charts and presentations.
Our Top FAQ's
Some common challenges faced when creating visualizations include:
-
- Finding the right balance between simplicity and clarity, and providing enough detail to convey the necessary information
- Choosing the appropriate type of visualization for the data and the message being conveyed
- Ensuring that the visualization is accurate and does not distort or mislead the interpretation of the data
- Making the visualization visually appealing and easy to understand for the intended audience
To overcome these challenges, it can be helpful to:
-
- Understand the limitations and potential biases of different visualization techniques
- Use clear, concise labels and captions to help convey the meaning of the visualization
- Use appropriate scales and axes to accurately represent the data
- Avoid using unnecessary visual elements or adding too much clutter to the visualization
When choosing the appropriate visualization for a given dataset, consider:
-
- The type and structure of the data being visualized
- The message you want to convey with the visualization
- The audience for the visualization and their level of familiarity with the data and subject matter
- The type of analysis you want to perform with the visualization (e.g., comparing values, identifying patterns, etc.)
To ensure that the design of a visualization does not distort or mislead the interpretation of the data, consider:
-
- Using accurate scales and axes
- Avoiding the use of unnecessary visual elements or adding too much clutter to the visualization
- Using clear, concise labels and captions to help convey the meaning of the visualization
- Ensuring that the visualization accurately represents the data and does not hide or distort important features or trends
Some best practices for creating clear and accessible visualizations for a diverse audience include:
-
- Using clear, concise labels and captions to help convey the meaning of the visualization
- Ensuring that the visualization is visually appealing and easy to understand
- Avoiding the use of unnecessary visual elements or adding too much clutter to the visualization
- Choosing colors and other design elements that are easily distinguishable and not confusing for the intended audience
- Ensuring that the visualization is accessible to people with disabilities, such as those who are colorblind or have low vision.